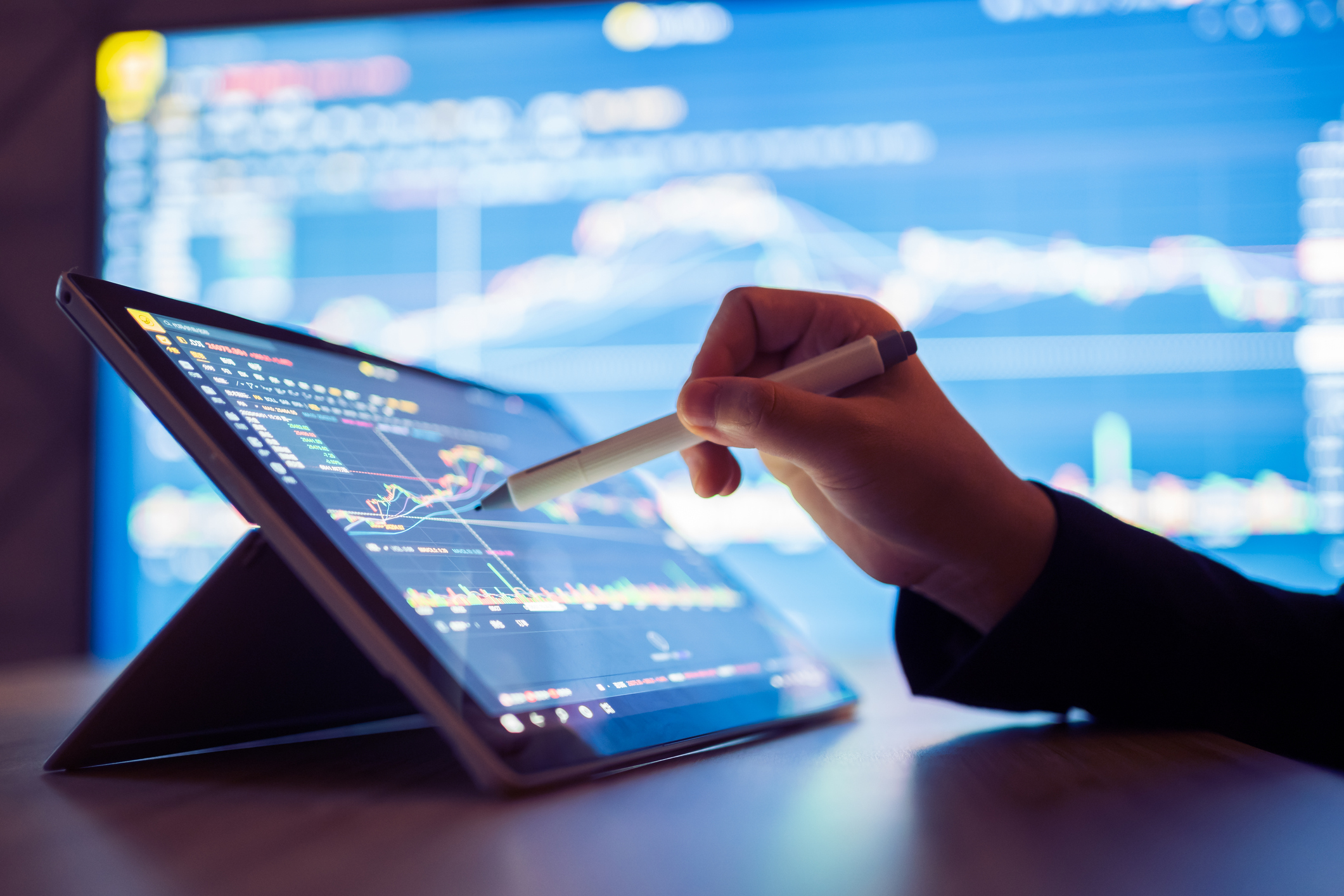
Policy GIGO – Living in a time of Noisy Data
The pandemic and its aftermath have thrust policymakers into dangerous waters. Policymakers are currently sailing through a data fog, in which extremes in data points continue to plague traditional mathematical models. These models guide their management of the economy and determine the best course of action.


Dr Michael P. Streatfield, CFA
Quantitative strategist, STANLIB Multi-Strategy
Key takeouts
- The path to normalisation of the global economy post-pandemic is still bumpy. This is evident in large extremes in macro variables, making it challenging for policymakers to forecast and make decisions.
- Due to this data fog, the data-driven Fed now plays a game of ‘wait and see’. It relies on actual, not forecast, data to guide policy decisions. Policymakers are also turning to alternative macro measures in attempting to penetrate the “noise”.
- Navigating with unreliable instruments (given this noisy data) and waiting for the last minute to turn (being data-driven) is amplifying the risk to markets of a policy mistake.
Overview
Models are built for “normal” times, and are not designed to handle noisy inputs. This presents challenges for confident forecasting and setting policy. Using a quantitative approach, we assess the extent of variability of macro-economic data, and find it remains a noisy environment, post-pandemic.
The US Federal Reserve, as we explained in April, is reacting differently to emerging data points. This new approach, macroeconomic and supply chain distortions, and current high transitory inflation makes it difficult for investors to anticipate the Fed’s next move, even when considering alternative Fed measures to gauge inflation.
This demanding forecasting environment raises the unfortunate risk of central banks making a policy mistake. Although this is not currently our base case, being aware of potential scenarios is a useful discipline. It ensures that investors are cognisant of regime shifts and prepared for portfolio action.
Sailing in a GIGO Fog
GIGO, or Garbage In, Garbage Out, is well known to quantitative experts (quants). It refers to the fact that poor data inputs into models will generally lead to nonsensical and poor outputs.
Data is the lifeblood of any modelling and quants will often “clean” their data to lower noise and improve their model fit to boost the accuracy of forecasting. Machine learning techniques also draw on this and refer to it as pre-processing the data. This process typically relies on the removal or trimming of extreme values – “winsorizing” is the jargon. It is acceptable to remove the odd outlier, given it could have resulted from a highly unusual time, or from poor data collection, and is likely to distort the outcome. However, these models and principles apply to normal times when data inputs are not at extremes.
Econometric models (statistical models of the economy) are important as they help decision-makers make sense of complex economic and financial systems. The US Central Bank, the Fed, has 400 PhD economists to help it to analyse economic data to guide timing of decisions, such as lowering interest rates to stimulate business growth.
To visualise how difficult it is for policymakers to navigate through the current waters, we looked at how many basic macro-economic variables, like growth, inflation and unemployment, were at year-on-year extremes. Extremes are measured by how far away from the average these data points are. One way of assessing this ‘distance’ from average is by using multiples of the standard deviations of data. Three standard deviations in a normal distribution correspond to a one in a 100 chance (so quite unlikely), and two standard deviations as a 5% chance.
Figure 1 shows the current high proportion of macro variables at extreme levels, as measured by those that are more than two or three times their standard deviation over the last five years. The large proportion of noisy data points illustrates that policyholders have truly been sailing through a fog in the last 18 months. They have no way to reliably expect that a large proportion of noisy variables put into existing quant models will give anything but garbage out. The word “unprecedented” has been over-used but it has certainly been such a time, as we have had to deal with global lockdowns, record stimulus packages, and working from home.

The response by policyholders like the Fed is to move from a reliance on forecasting models to a game of “wait and see”. Essentially they are waiting to see what the actual data point is. The Fed has also added some softer, less “quantitative”, more qualitative measures to its agenda, which adds to forecasting difficulties (as outlined in the “Not your grandfather’s Fed” piece written in April. 2021). Fed Chair Jerome Powell, in the recent Jackson Hole Conference in August 2021, re-iterated its data-driven approach of “carefully assessing incoming data and the evolving risks”.[1] The danger of this approach is like plotting a course but waiting to see the reef before you touch the helm to act.
It is not only central bankers who find it difficult to forecast in this environment. Private sector economists aim to guide investment decision-making. We have observed that the range of inflation and growth forecasts by market economists continues to remain far wider than pre-pandemic levels. For example, the 2021 US real GDP forecast range between high and low estimates among 83 economists is more than double the 2018 range. Model risk thus remains high, and confidence in the true state of the trajectory of the economy remains low.
Don’t take at face value what I mean
It is one thing for policymakers to be “data-driven”. The challenge becomes knowing which data drives their decisions, as the system swells with noise, and acknowledging that even then, policymakers have created enough room in their ambitions to “justify” any inaction or not.
The Fed’s favourite measure of inflation is not CPI, but the usually staid Core “PCE” (Personal Consumption Expenditure). PCE is seen to be more reflective of all consumption prices in the economy, not just those to which consumers are exposed. To moderate this further, the Fed looks at Core PCE, which excludes food and energy, the noisier parts of the inflation basket. This gives the Fed a fairly stable, balanced measure to assess inflation pressure in the US economy.[2]
It all sounds good in theory, but the current distortions of transitory inflation are so large that even central bankers are looking to move the yardstick (or goalposts). New alternative measures are being explored. For example, the Dallas Fed Trimmed Mean PCE measure, which lops off the biggest price rises and smallest price falls, or the Cleveland Median PCE, which looks at the middle of a set of changes.

In Figure 2, even after “adjusting” inflation to the less noisy measures (trimmed or median), we are still seeing an uptick in inflation. All of these datapoints exceed the Fed’s historical response trigger level of 2%. This presents a conundrum for investors. Do they simply wait for this so-called transitory data fog to pass, or do they look for meaning behind these new measures and means?
Rise of the Risk of a Policy Mistake
In April we warned in our Not your Grandfather’s Fed piece that the Fed had changed its reaction function, and would be more patient with inflation overshoots. It would choose to focus more on the employment recovery.[3] The Fed has stuck to its Average Inflation Targeting message, which broadly says it will aim to achieve an average inflation of 2% over time, and allow for it being above 2% for some time.
The brief recession, resulting from the pandemic-induced temporary closure of the economy, has certainly created base effects which amplify the noise in the system. However, jumpstarting the global economy is proving harder than expected. Hotels, rental cars and airline tickets have shown temporary large surges in prices. The automobile sector has suffered severe semi-conductor chip shortages. Chips in automobiles are only a very small part of the average car value, yet these necessary components – with few substitutes – have limited car production. IHS has estimated lost production of eight million cars in 2021 (5% of the light passenger market). Autos are a small player, at 8%, in the global chip market, so they stood at the back of the queue, well behind consumer electronics products. Investors should expect problems to persist. For example, VW and Daimler Mercedes have guided investors that these disruptions could last beyond next year to 2023.[4]
Supply chains are only as strong as their weakest link, which could alter the transitory narrative to which central banks are clinging. Potential rolling waves of disruptions could maintain these price and data distortions beyond 2021. Note this is only one risk and it ignores geopolitical risks, a China hard landing, or new, more dangerous COVID-19 variants that will also keep the data rollercoaster going.
Sailing in a storm is one thing, but navigating with unreliable instruments (given this noisy data) and waiting for the last minute to turn (being data-driven) is amplifying risks for the financial system. Although it is not our base case, there is rising concern that central bankers risk making a policy mistake as they move to normalise huge levels of market stimulus. This raises the level of market risk at a time when lower yields have raised reliance on equity in portfolios.
This policy risk is complex and can have more than one bearing. On one side there is the risk that policymakers become spooked by transitory inflation lingering for many quarters, as this may start to feed into escalating inflation expectations and wage rises. Central bankers may then choose to pre-emptively raise interest rates, the only tool they possess to tame rising inflation. This could be devastating at a time when the global economy is still trying to recover, because of the flow-through of higher interest costs, given the high levels of indebtedness at both corporates and governments, and the convexity impact when rates are low. These concerns could lead to a stampede from risk assets.
On the other hand, there is the risk that policymakers take no action, as they continue to focus on unemployment without anticipating the extent of the structural impact of the pandemic/endemic. The stubborn high levels of unemployment could be due to skill mismatches, and, perhaps, expectations of further stimulus support. Skill mismatches will take time to resolve, as people re-train. Continued helicopter money “support” during this period could lead to even more excessive risk-taking by market participants and fuel bubble behaviour.
Implications for Investors
Investors worried about policy risk may chose to de-risk and “move to cash” but it is important to remember that markets are always faced with threats. Investors who moved to cash at the start of the pandemic would have lost out on any subsequent returns during the rapid recovery in markets. Our approach in the STANLIB Multi-Strategy team is to think through the “what might be’s”, using scenario analysis.
By thinking what potential scenarios investors could face over the next 12 months, we prepare and think through asset class behaviour in each scenario. Not all scenarios are equally likely, but this exercise has a number of advantages:
- Keeps an open mind: You are not sucked into the prevailing Goldilocks narrative.
- Early warning system: You start to scan the news flow for precursors to one of these scenarios, so you can be ready for a market shift.
- Anchoring: We tackle the behavioural finance bias of anchoring to the existing portfolio view, and ‘explaining away’ any disconfirming evidence as it emerges.
- Action plan: If the regime does shift, then the team has already thought through some of the asset class impacts in advance. This helps the portfolio to make the transition and speeds up execution for our investors.
It is impossible to predict what will happen, but being prepared is an essential element in multi-asset investing. Hoping for the best, but expecting the worst, is an even more valuable skill in this time of higher data distortions.
[1] Powell’s speech “Monetary Policy in the Time of COVID”, 27 August 2021, can be found here: https://www.federalreserve.gov/newsevents/speech/powell20210827a.htm
[2] For example, PCE has a more balanced weight to housing (22%) than the US CPI basket (42%).
[3] See https://stanlib.com/2021/04/22/not-your-grandfathers-fed/
[4] See more on this story at https://www.bloomberg.com/news/articles/2021-09-05/mercedes-boss-warns-chip-shortage-won-t-just-go-away-next-year